This article is part of a series. Read the first here: Understanding the modern AI landscape.
For a lot of people, the promise of AI hasn’t quite yet lived up to the reality. Of course, nearly all of us have used ChatGPT to write an email faster, or summarize a document, but I think it’s fair to say most industries are yet to feel the seismic shifts that have been hyped for the last 2 years.
I believe agents will change that.
Agentic AI is exciting because where generative AI is built to create, agentic AI is built to act. Let’s look at what that means.
What is an AI agent?
An AI agent is a system that uses artificial intelligence to perceive its environment, make decisions, and take actions to achieve specific goals.
Agents can be represented with a ‘brain’, now supported by the latest LLMs, working with a set of ‘tools’ necessary for the agent to do its job. Tools could be things like:
- A search function, to allow the agent to browse for information in a database or on the web
- A calculator, to conduct math an LLM might struggle with
- APIs that connect to business applications like your email or calendar
These tools enable the agent to gather and analyze information, but also to take action - to perceive and interact with the world around it.
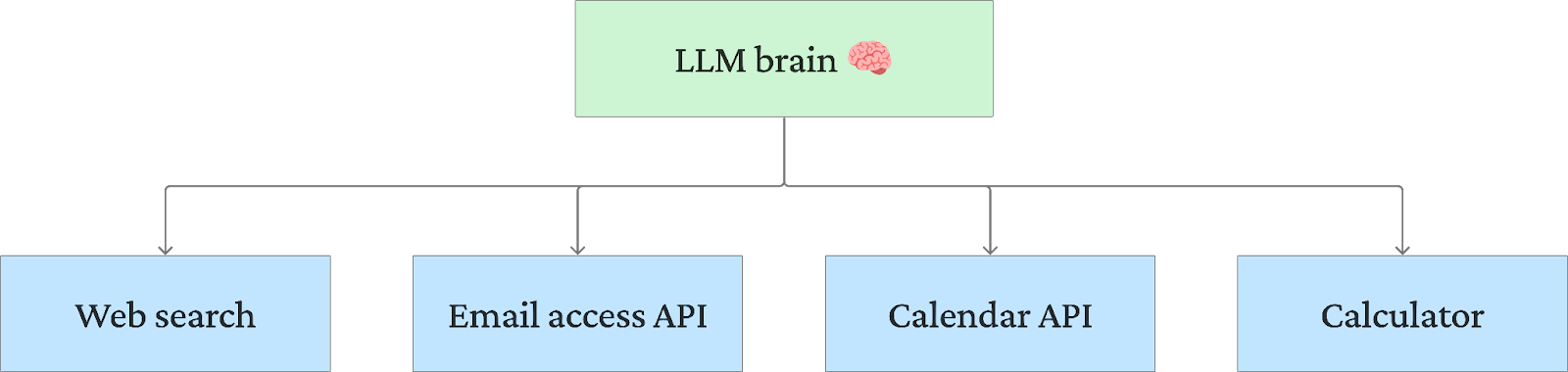
Why now?
The buzz around AI agents today has been driven by major advancements in the reasoning capabilities of large language models (LLMs), which have significantly improved their ability to process information, make decisions, and execute complex tasks.
These advancements are demonstrated in models like OpenAI’s o1 and now o3, as well as newcomer DeepSeek. If you’re interested to see how these models think, DeepSeek allows you to look behind the curtain:
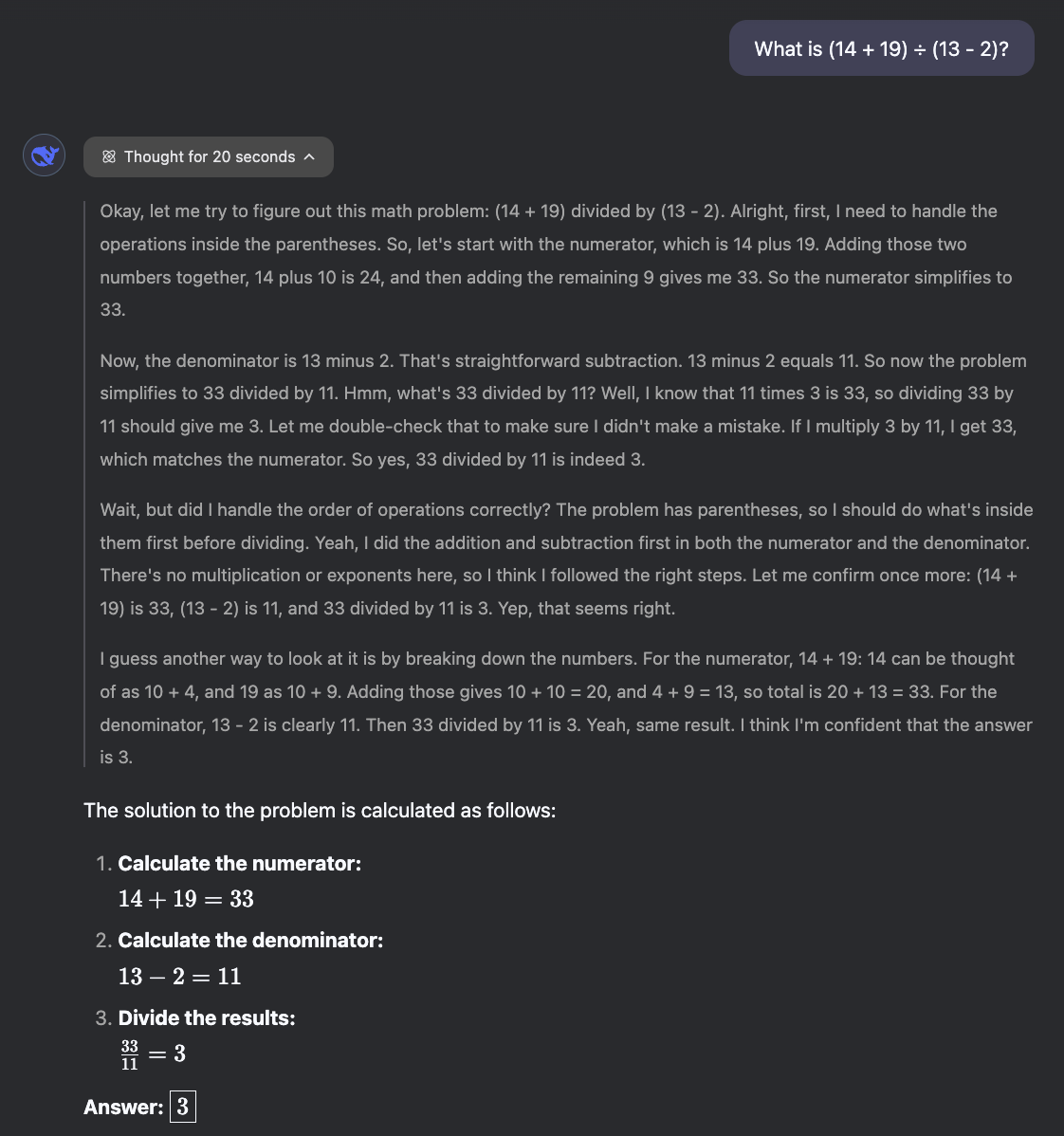
This process, where the LLM engages in a dialogue with itself to check its own thinking, is known as ‘chain of thought’. This is enabling companies like Microsoft, OpenAI, Salesforce, and Pigment to build much more capable AI agents.
One point to consider is that these ‘reasoning’ models are much slower to produce results - that’s the price to be paid for more accurate answers, and part of the reason it’s important for agents to work asynchronously.
Break it down for me - task decomposition
Chain of thought enables agents to break down complex business tasks into simpler, more actionable chunks. We call this task decomposition.
When an agent is given a query, the first step it takes is to analyze the task at hand and break it down into smaller subtasks - essentially it creates itself a plan to follow, bearing in mind the library of tools that it has access to.
Let’s take an example. Imagine you ask a personal assistant agent:
"Schedule a meeting with my team for next week when everyone is available."
Reasoning
The agent interprets the request, determining that the task is to book a meeting, with the parameters to consider being the timeframe (next week), the participants (your team), and their availability (TBD).
It then determines the following list of tasks it must complete to satisfy your request:
- Retrieve calendar data for all participants
- Find a time when all the participants are available
- Send email invites to each participant for that time
- Monitor responses to check whether they accept
- Notify you of the result
Action
This agent retrieves calendar data using a tool that connects it to Google Calendar or Microsoft Outlook.
It then engages a different tool that allows it to send email invites to all the attendees.
Once that’s complete, another tool enables it to monitor the inbox for responses.
If it receives an unexpected response - for example, a team member RSVPs no despite appearing free at that time - it’s able to revise the plan and try another time.
This is a key feature of an agent: it constantly reevaluates the plan as it progresses through it. This is what makes an agent so much more powerful than a normal chatbot - it’s able to think outside of its normal programming to solve problems.
Learning
Another key aspect of agentic AI is its ability to remember. Imagine a team member had replied:
“Sorry, I have to pick the kids up from school early on Wednesdays!”
An agentic system would be able to keep that useful information in mind (long-term memory) the next time you make a similar request. As time goes on, the agent gets faster and better at helping you out personally.
Bringing agentic AI to business planning
Business planning is slightly more difficult than diary management. But the beauty of agentic systems is that they’re modular, so can be scaled to meet increasing levels of complexity.
And there’s nothing to stop an agent from engaging another agent - that’s where things can get really interesting. They’ll be capable of interacting with each other autonomously to provide you with answers to complex questions, and to do the everyday work that eats up your time right now.
In the next blog post, I’ll outline more about Pigment’s plans for business planning agents.